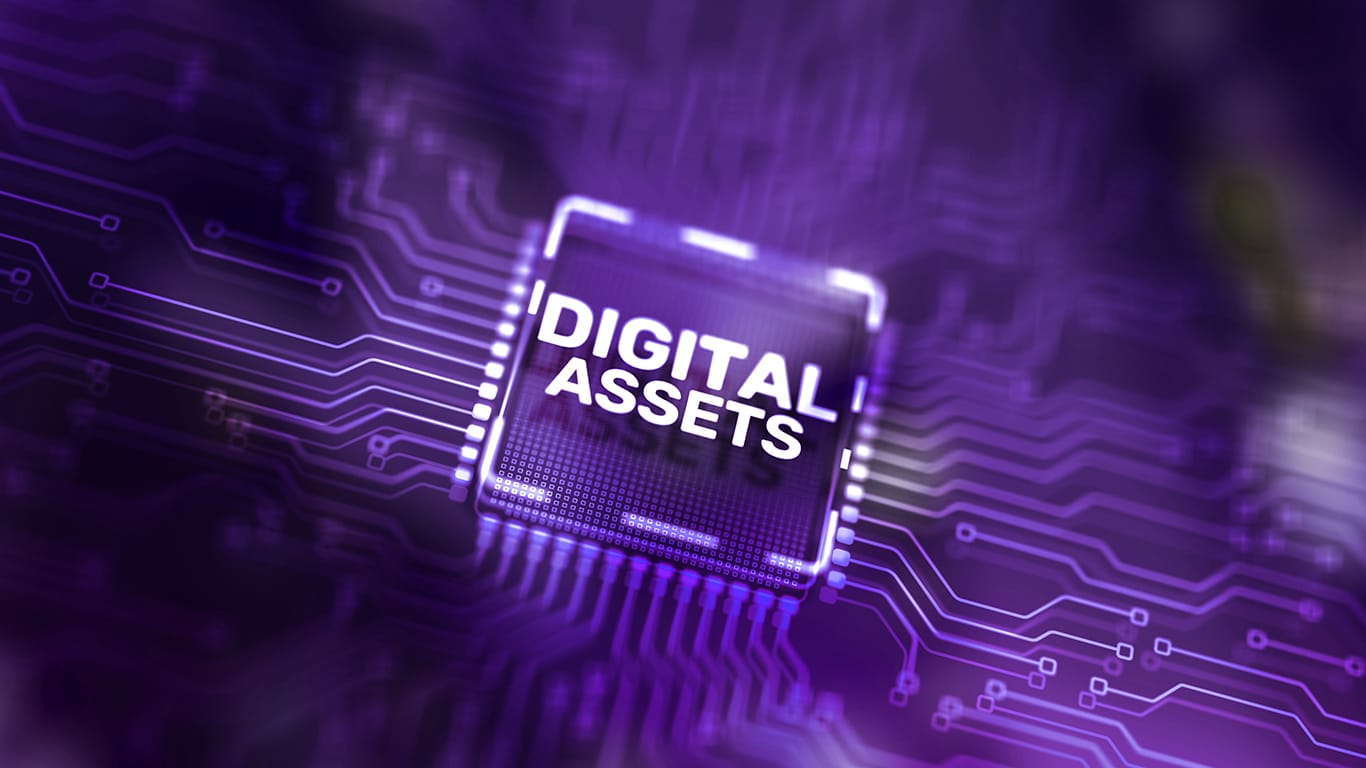
Frequently Asked Questions about Digital Asset Management
Here, we delve into the complexities and nuances of managing vast digital assets in today’s fast-paced digital environment. As a leader in data management solutions, Rimage brings clarity to the intricacies of DAM, providing insights that help organizations optimize their digital workflows and enhance data security.
Our FAQ was developed to guide decision-makers and IT professionals looking to improve their digital asset management strategies. Whether you’re evaluating the benefits of on-premise versus cloud-based solutions, grappling with the challenges of dynamic data, or exploring the integration of AI within your DAM systems, this resource offers valuable information to guide your decisions.
At Rimage, we understand the critical role that effective digital asset management plays in a company’s operational efficiency and competitiveness. We aim to address common concerns, dispel myths, and outline strategic approaches to managing digital content securely and efficiently. Join us as we explore how to harness the power of DAM to transform your business operations and drive success.
______________________________________________________________________
Introduction to Digital Asset Management
What is digital asset management?
A digital asset management (DAM) solution simplifies your approach to global data management and preservation. When done right, it seamlessly automates data ingestion from any source and builds a global repository to organize and optimize digital assets in a streamlined and secure way.
Key Challenges in Digital Asset Management
What makes digital asset management difficult?
Organizations often struggle with digital asset management because the sheer volume and variety of data can overwhelm existing systems. Managing vast amounts of data requires sophisticated tools and strategies that can scale effectively.
Why are existing systems overwhelmed by large volumes of data?
Many traditional DAM systems are not designed to handle the scale and complexity of data generated today. They may lack advanced features like AI-driven automation and integration capabilities, which are essential for managing large datasets efficiently.
Why do traditional DAM systems lack the necessary features?
Initially, DAM systems were designed for simpler, less voluminous data sets and did not anticipate the explosive growth of data types, sources, and volumes. The rapid evolution of digital content production – which will only continue to increase with the adoption of AI – and the emergence of new data types have outpaced the development of many traditional management tools.
Evolution of Data and DAM Systems
Why did the evolution of data outpace DAM system development?
The exponential increase in data creation is largely fueled by advancements in digital technologies and the internet. Industries began leveraging digital platforms for a broader range of functions without concurrent advances in data management technology to support these new uses.
Why haven’t data management technologies kept up with advances in digital transformation?
Investment and innovation in data management technologies were initially not prioritized to the extent of data creation tools and platforms. There was a lag in recognizing the critical need for robust data management as an integral part of digital transformation strategies.
Choosing the Right DAM Solution
What should you consider when choosing between on-premise and cloud-based DAM solutions?
On-premise DAM solutions generally require a higher upfront cost, while cloud-based solutions bring recurring costs that may increase as data scales. Cloud solutions offer inherent scalability, but this can become costly as your data needs expand. On-premise solutions provide more stringent control over security, which is crucial for meeting compliance requirements. They also ensure complete control and ownership of data, eliminating risks like vendor lock-in or unexpected changes in service terms. A hybrid approach can balance cost, control, and scalability but requires effective management of both environments.
Managing Long-Term Access to Dynamic Data
What is dynamic data and why does it require a unique storage approach?
Dynamic data refers to information that frequently changes or updates over time due to user interaction or automated processes. Unlike static data, which remains unchanged unless manually altered, dynamic data continually evolves, often in real time. This characteristic makes it essential for organizations to have robust management strategies to ensure both immediate accessibility and long-term preservation. Examples of dynamic data include:
- E-commerce Inventory Levels
- Financial Market Data
- User Activity Logs
- Sensor Data from IoT Devices
- Content Streaming Statistics
How should dynamic data be managed for long-term access?
Managing dynamic data for long-term access is effectively done through a tiered storage approach, accommodating varying access and retention requirements. The initially higher costs of on-premise solutions might be offset by avoiding the ongoing costs of cloud storage. Employing various storage technologies, such as SSDs, LTO, and high-capacity optical disks, based on data usage and longevity, can optimize costs and accessibility.
Enhancing DAM Efficiency Through Integration
How can integration with collaboration tools enhance DAM efficiency?
Integrating DAM with project management and other collaboration tools centralizes data interactions and automates workflows, enhancing efficiency and ensuring a single source of truth. This integration also allows for advanced metadata management, improving asset discoverability and automating data lifecycle management. Additionally, it enhances data insights from integrated systems, which improves decision-making around asset use, management, and cost optimization.
What are the considerations for using AI within DAM, particularly with sensitive or regulated data?
While AI offers significant automation benefits in data management, its use must be carefully managed when dealing with sensitive or regulated data. Employing purpose-built AI in controlled environments allows for better management of data inputs and model training, reducing risks of bias and errors. Starting with small, manageable AI applications helps control risks and allows for gradual expansion as confidence and systems mature. This approach ensures that AI is used ethically and effectively within DAM systems.
Tips to Successfully Implement a DAM
- Adopt AI-Driven DAM Systems: Implementing modern DAM solutions that leverage artificial intelligence can help automate tasks such as data tagging, categorization, and analysis, making the management of large data sets more feasible and efficient.
- Enhance System Integration: Upgrade DAM systems to ensure they can integrate seamlessly with other tools and platforms used within the organization, enhancing the flow and accessibility of data across different departments.
- Continuous Innovation and Investment: Organizations should continually invest in and update their DAM strategies and systems to keep pace with technological advancements and the evolving landscape of digital data.
- Tailored Solutions for Big Data: Develop or adopt DAM solutions specifically designed to handle big data environments, focusing on scalability, security, and speed to manage large volumes of data effectively.
- Educational and Training Initiatives: Implement training programs for IT staff and users on the capabilities and utilization of advanced DAM systems to maximize their potential benefits for the organization.
Want more tips? Download our DAM Checklist – a comprehensive guide to selecting, implementing, and maintaining a digital asset management solution to transform your data into a valuable organizational asset.